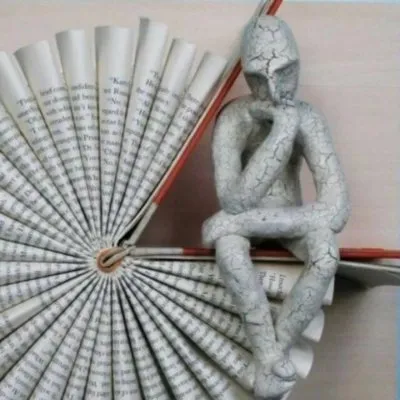
Haotian | CryptoInsight|Apr 23, 2025 11:33
As is well known, the MCP protocol has not only sparked a significant wave of agent linkage in the web2 AI field, but is also penetrating towards the web3 AI direction to find a foothold. However, for web3 AI agents to solve the problem of application implementation, besides the MCP protocol, how to solve the problem of data memory gap is the most urgent. Here is a solution provided by @ MemopoAi:
1) Memo Protocol is positioned as an agent platform that integrates AI and blockchain, attempting to build a unified standard Knowledge Base for web3 AI agents. In other words, it is necessary to transform the chaotic and unstructured data on the chain into an effective data source that can be directly called by the agent, becoming a necessary component for the agent to land in vertical application scenarios.
As described below, the first web3 knowledge set currently available includes the On chain Knowledge Base Token Insights、 Yield Navigator, Allow users to personalize the liquidity data profile of their wallets and smart contracts and apply it to investment capture high APY return pools.
I won't go into detail here. Users can actually access and experience it, especially AI agent developers can try deploying integration to verify the feasibility of their data service solutions.
2) I will mainly share some innovative points I have seen from the perspectives of technology and economic model design:
1. PPR+topology monitoring algorithm: Compared with the simple similarity matching of traditional vector databases, PPR (Personalized PageRank) algorithm can better capture the correlation and hierarchical structure between knowledge, which is closer to human knowledge organization. Topology monitoring enables knowledge networks to perceive and adapt to new information in real-time, maintaining their "activity";
2. Dynamic Graph Storage Engine: Unlike static knowledge graphs, Memo achieves dynamic fusion and evolution of cross domain knowledge, enabling AI agents to perform more complex reasoning (such as association analysis between medical risks and financial investments), and the project's Cofounder is an expert in graph databases at Nanyang Technological University;
3. Zero Knowledge Proof (ZKP) mechanism: Achieving controllable knowledge sharing while protecting privacy, which is particularly important for sensitive fields such as healthcare and law.
4. PoKC (Proof of Knowledge Contribution) mechanism for quantifying knowledge contribution: By quantifying the value of knowledge contribution, it solves the problem of "data exploitation" in traditional centralized API platforms, allowing knowledge creators to receive fair rewards. Subsequently, a self reinforcing ecological cycle is formed, consisting of knowledge miners (contributing professional knowledge), agent developers (consuming knowledge), and node operators (maintaining the network).
3) After understanding the product and technical features, returning to the question mentioned at the beginning, why does web3 AI need a completely new infrastructure layer? The reason is also very simple:
The current development of AI agents faces a fundamental contradiction: the more specialized the agent, the higher the knowledge barrier; More general agents lack professional depth. This has led to three core pain points:
1. Knowledge island phenomenon (fragmentation): Agents from different vertical domains are unable to effectively share knowledge, such as medical agents being unable to directly call financial data for cross domain reasoning;
2. Transient memory (stateless): Most agents rely on real-time retrieval (RAG) and lack persistent memory mechanisms, making it difficult to form continuous output results;
3. Repetitive construction (without sharing mechanism): Each field needs to build a knowledge base from scratch, which greatly wastes resources and reduces innovation efficiency;
Obviously, the MCP protocol can solve some of the islanding and redundant construction problems, but there is no urgent need for real-time data analysis and calling required by web3 DeFai, GameFai and other scenarios in the web2 AI scenario. Therefore, there is not a significant need for data state storage. To implement web3 AI agents, the first challenge is to build an effective data layer of Infra.
above.
Finally, based on the above sharing, the development strategy for the web3 AI Agent track can be summarized as: avoiding a direct confrontation with web2 AI in application implementation, and focusing on building the unique infrastructure layer of web3 AI. Accelerating the layout in this niche track is the key path for the differentiated development of web3 AI agents.
Share To
HotFlash
APP
X
Telegram
CopyLink