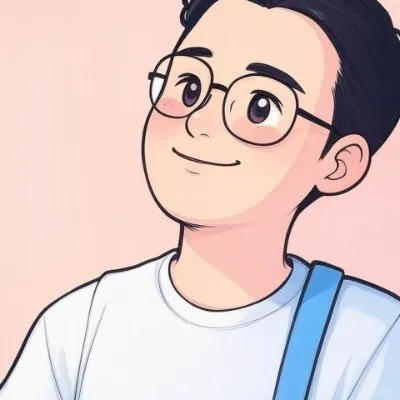
NingNing|Mar 12, 2025 06:30
TGE Spring is accelerating at full speed! Will the market's FUD sentiment towards VC incubated new coins dissipate, and the narrative of cryptocurrency technology take advantage of this opportunity to experience a wave of Renaissance?
In the 100% orthodox encryption technology narrative ZK (zero knowledge proof) and FHE (fully homomorphic encryption), ZK has been fully priced by the secondary market, while FHE is still in the overvalued bubble of the primary market. For example, Zama completed a second round of funding of $73 million in March 2024, and Mind Network completed a Pre-A round of funding of $10 million in September 2024.
Against the backdrop of all projects accelerating TGE in Q1 of the 25th year, Mind Network, which has entered the Pre TGE stage, is expected to become the first FHE project to take risks. It's time for the secondary market to test the quality of FHE's slightly divine technological narrative in the primary market.
one ️⃣ The basic principle of the 72 variable FH of the grid
The technical principles of ZK and FHE are both very hardcore and abstract (literally abstract), but through Vitalik's small papers, VC agency investment reports, and KOL Thread's year-round carpet bombing science popularization, many people inside and outside the circle understand some basic concepts, such as the classic "verify as true without revealing any information". Compared to ZK, FHE's market awareness is more than one level lower.
The mathematical principle of FHE technology is to use a lattice based encryption scheme to perform arbitrary calculations on encrypted data without leaking the original data through homomorphic mapping and noise management.
Lattice is an important concept in mathematics, widely used in fields such as algebra, number theory, and cryptography. Simply put, a lattice is a discrete set of points generated by a set of basis vectors, which are arranged in a periodic manner in the vector space. The security of FHE usually relies on lattice learning with error problems (LWE), etc.
The specific method of utilizing lattice structure in FHE schemes (such as BGV, BFV, CKKS, etc.) is as follows:
🔹 Encryption mapping: Map plaintext to points in a lattice, and then add an appropriate amount of "noise" to hide the true value.
🔹 Homomorphic operations: The addition and multiplication of encrypted data correspond to operations on lattices, but these operations add noise.
🔹 Noise management: The key challenge of FHE is managing the noise growth during the operation process, which is typically addressed through techniques such as "bootstrapping".
🔹 Polynomial representation on the ring: In practical implementation, the elements on the polynomial ring are usually used to represent lattice points to improve efficiency.
Simply put, FHE allows for direct computation of encrypted data without the need for decryption, with the entire process from data acquisition, transmission to computation being in an encrypted state.
FHE and ZK are listed as two holy grails in the field of encryption, but each has its own focus:
🔰 FHE focuses on data privacy: encrypted data can still be computed, suitable for cloud computing and AI processing of sensitive data;
🔰 ZK focuses on proof rather than computation: proving something without revealing additional information, suitable for identity verification and privacy transactions.
The practical application scenarios of FHE mainly include privacy training of LLM models in the AI field, blockchain privacy cross chain and anti MEV, and Multi Agent security consensus frameworks.
two ️⃣ Paradigm Fusion - Mid Netwrok's Supply Side Solution
After popularizing the basic principles of FHE, let's focus on analyzing the first TGE project of the track, Mind Netwrok.
The Mind Network project is located at T1 of the FHE track, and is committed to the realization of Zero Trust Internet Transmission Protocol (HTTPZ). It is mainly aimed at AI privacy and security, and has received investment from well-known institutions such as Binance Labs, Hashkey, Chainlink, and two grants from the Ethereum Foundation.
The architecture design and operational strategy of Mind Network have broken the stereotype of encryption geek teams in the market. They really understand Web3 and are very good at organizing work.
On the supply side, Mind Network optimizes FHE computing efficiency with industry leaders such as Zama's advanced FHE technology stack and hardware acceleration solutions, while reducing FHE computing costs through the design of decentralized network structures and token economics.
In the Zama team, Paillier is the inventor of the Paillier cipher and currently the most commonly used algorithm for additive homomorphic encryption. The open-source FHE tools provided by ZAMA, such as Concrete ML and fhEVM, are currently the most commonly used open-source libraries. They have collaborated closely with Mind Network to simplify the computation process for developers in encrypting data, serving fields such as AI and Web3. The core solution of Mind Network for the AI field is MindChain, which is also the first FHE Chain serving AI agents. AI agents are currently at the forefront of Web3 and AI fields, but agents often face more security risks in decentralized environments. MindChain provides end-to-end encrypted verifiable computing based on FHE, allowing AI agents to maintain security in areas such as information exchange, data storage, and collaborative decision-making without exposing underlying data.
In addition, MindNetwork has also launched MindLake, a Rollup solution for data storage and privacy computing based on FHE. And provide services for decentralized storage networks such as Arweave, IPFS, and BNB Greenfield. On the other hand, in the field of decentralized AI, such as distributed GPU computing and machine learning protocols, Mind Network also collaborates with platforms such as Bittensor to help provide trusted and fair encryption computing mechanisms, promoting progress in the decentralized AI field.
three ️⃣ Scenarios and Flower Activities - Demand Side Operations of Mid Netwrok
On the specific AI demand side, Mind Network has discovered four major landing scenarios that fit the constraints of the reality of the encryption industry:
Scenario 1: AI Secure Computing - Safe Cockpit for Sensitive Data 🤖
The collaboration between Mind Network and DeepSeek reveals its primary application scenario: trusted AI. In traditional AI model training, data must be processed in plaintext form, which poses a risk of sensitive information exposure. Through FHE technology, Mind Network has achieved:
🔹 Encryption data training: Medical institutions can share data to train disease diagnosis models while protecting patient privacy
🔹 Privacy inference service: Financial institutions can use customer encrypted data for credit evaluation without accessing raw information
🔹 Multi party secure collaboration: Collaborative computation of data between different organizations without compromising data ownership
This scenario directly addresses the core pain points of the current AI industry: data silos and privacy concerns. DeepSeek integrates Mind Network's FHE Rust SDK to enable its open-source models to perform computations in encrypted form, expanding the application space for privacy sensitive industries such as healthcare and finance.
Scenario 2: Security Consensus Framework for Multi Agent Systems 🔄
As AI Agent evolves from a single model to a Multi Agent System, such as Swarms, Mind Network's AgentConnect Hub provides key infrastructure:
🟦 Security Agent Identity System: Provides decentralized identity (DID) for AI agents to ensure trusted interactions
🟦 Encryption consensus mechanism: Multiple AI agents reach consensus without sharing raw data
🟦 Verifiable AI decisions: The calculation results executed through FHE can be validated, enhancing system trust
This application scenario is particularly targeted at Multi Agent systems such as Swarms, enabling AI agents to work collaboratively while protecting their respective data. Imagine a medical diagnosis scenario: multiple specialized AI agents analyze the same encrypted patient data to jointly form a diagnostic opinion, while the patient data is always in an encrypted state.
Scenario 3: Cross chain asset security bridging 🌉
Mind Network's FHE Bridge collaborates with Chainlink to provide a new paradigm for cross chain interaction:
🟦 Cross chain transmission: Keep assets fully encrypted during the process of cross chain transfer
🟦 Self verification: The receiving chain can directly verify the validity of encrypted assets without the need to trust third parties
🟦 Anti censorship transactions: The transaction content is kept private throughout the entire process, reducing the risk of MEV attacks and censorship
Unlike traditional cross chain bridges, FHE based bridges allow for verifying the validity of cross chain transactions without decryption. This provides higher security for DeFi protocols and cross chain applications, especially when handling large transactions.
With a stage, who will sing the opera? On the one hand, Mind Network has activated 1.78 million active wallets and 1 million community members through CitizenZ's mainnet points campaign, and has already tokenized over $2 billion in assets on its platform; On the other hand, it collaborates with projects such as Eigenlayer, decentralized GPU computing power networks, open-source big models, AI Agent framework projects, and democratized AGI in the field of AI to capture real B-end requirements for its FHE services.
For example, DeepSeek, the king of open-source big models, has integrated Mind Network's FHE Rust SDK, which will allow AI agents based on DeepSeek to perform calculations under fully homomorphic encryption, ensuring data remains encrypted and secure.
For example, Mind Network has established partnerships with AI Agent framework projects such as Swarms, ai16Z, and Viturals, providing them with Multi Agent systems, FHE+TEE, and encryption inference services and support based on FHE. Mind Network plans to provide FHE security services for over 3000 AI agents in the future.
four ️⃣ summarize
It has been a long time since there was such a white horse asset in the secondary market that combines authentic encryption technology narrative, trendy architecture paradigm, and down-to-earth project operation. The arrival of Mind Network may guide the main logic of the secondary market to return from "excessive casino like" to "narrative of encryption technology".
But we must also candidly admit that FHE is on the left side of the emerging technology curve compared to ZK. At present, the implementation of FHE technology still faces significant challenges, including:
🔹 Performance threshold: Even after optimization, FHE computation overhead is still much higher than plaintext computation
🔹 Development complexity: FHE application development requires professional cryptographic knowledge, with a steep learning curve
🔹 Lack of standardization: The industry lacks a unified FHE standard, and interoperability between different implementations is limited
So, currently we cannot use the business valuation model of real protocol revenue to value Mind Network. A reasonable approach is to discount the future value of FHE technology in the present. Of course, there is a complex reflexive relationship between future value and current price. When simple mathematical models are unable to handle this, we can refer more to the pricing in the pre trading market.
above.
Share To
Timeline
HotFlash
APP
X
Telegram
CopyLink